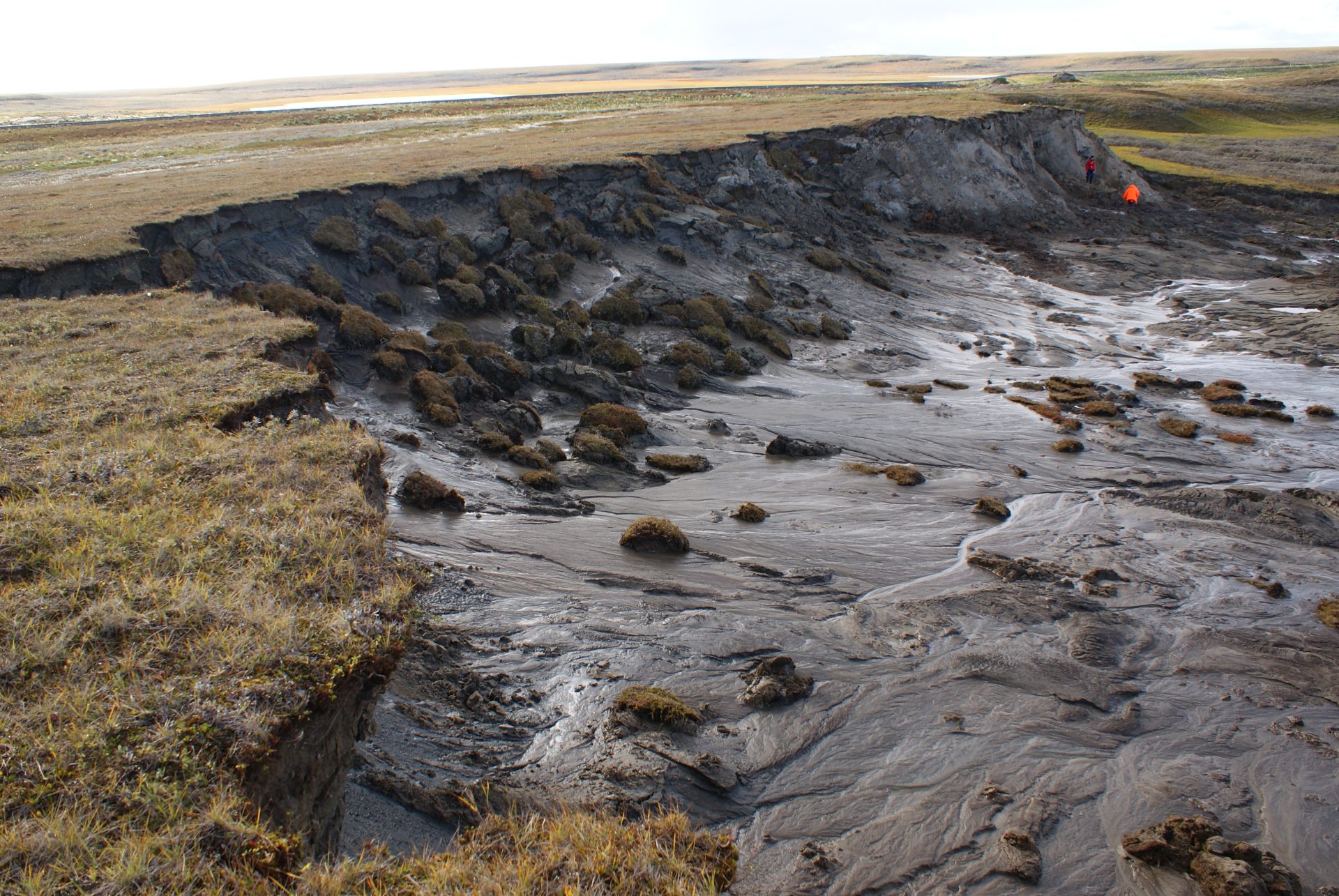
©Annett Bartsch (25.08.2015)
Earth system data consists of heterogeneous, big datasets encoding not fully understood spatio-temporal geophysical and atmospheric processes. These complex datasets pose unique challenges to Earth scientists decoding natural processes to solve global environmental problems. Recently, algorithmic developments and impressing capabilities of artificial intelligence led to first real world applications using environmental data.
KI:STE aims to facilitate the application of large scale machine learning on high performance computing (HPC) systems for Earth system data. It uses a sophisticated strategy combining development of
an user-friendly Earth-AI platform with an Earth-AI e-Learning platform.
These two platforms are based on the scientific insights, requirements, experiences, challenges and resulting machine learning workflows. The Earth-AI platform will create the technical prerequisites to make HP AI applications on environmental data portable for future users.
Within the KI:STE project, five doctoral researchers tackle Earth system research questions using data-driven approaches. A novel approach of mapping the probability of shallow landslides is tackled using heterogeneous data, explainable machine learning and uncertainty quantification. Moreover, based on land cover classification by a deep neural network, the notion of wilderness as captured by the model is explained using explainable AI techniques. State-of-the-Art unsupervised machine learning approaches are used to classify clouds and vegetation from satellite images. In addition, to improve predictions of numerical hydrological models, deep learning for error correction is under development.
The KI:STE consortium consists of academic and industrial partners. The collaborating partners are University of Cologne, University of Bonn, RWTH Aachen University, Ambrosys GmbH, 52°North GmbH and Forschungszentrum Jülich. The excellent education of our doctoral researchers is supported by the graduate School HDS-LEE.
Funding for the KI:STE project is provided by the Federal Ministry for the Environment, Nature Conservation and Nuclear Safety and supported by Z.U.G.. The grant is valid for the period from 01.11.2020 to 31.10.2023. Grant Agreement: 67KI2043.